Investment Strategies
Psychological Aspects of Trading: Mastering Emotions for Financial Success
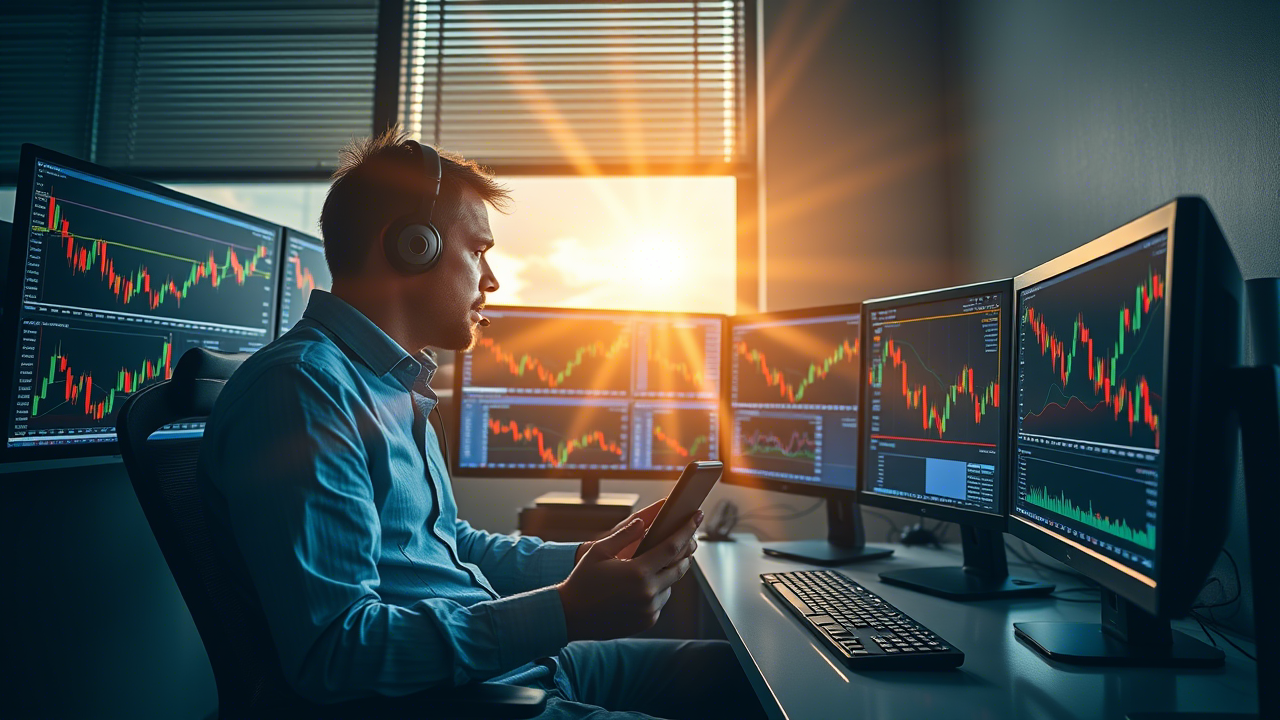

Psychological Aspects of Trading
Trading, whether in stocks, forex, or cryptocurrencies, is as much a psychological endeavor as it is a financial one. The market is a dynamic and unpredictable environment, and your emotional state can significantly impact your trading decisions and outcomes. Understanding and mastering the psychological aspects of trading is essential for anyone looking to achieve long-term success. In this article, we will delve into the emotional challenges traders face, provide current data on the psychological impact of trading, and offer practical tips to help you develop a more resilient and effective trading mindset.
The Emotional Rollercoaster of Trading
Trading is often associated with high levels of stress and emotional turbulence. The constant fluctuations in market prices can trigger a range of emotions, from euphoria and excitement to fear and despair. These emotional responses can be powerful and, if left unchecked, can lead to impulsive decisions that undermine your trading strategy.
Common Emotional Pitfalls
- Fear: The fear of losing money can paralyze traders, causing them to hesitate in executing trades or to close positions prematurely.
- Greed: The desire for quick profits can lead to overtrading and taking on excessive risk.
- Hope: Hope can blind traders to the realities of the market, causing them to hold onto losing positions longer than they should.
- Overconfidence: Success can breed overconfidence, leading to complacency and poor risk management.
- Desperation: Repeated losses can create a sense of desperation, causing traders to make irrational decisions in an attempt to recoup their losses.
The Impact of Emotions on Trading Performance
Emotions can significantly influence trading performance, often leading to suboptimal decisions. According to a study by the Journal of Behavioral Finance, emotional decision-making can result in higher trading costs, increased risk of losses, and lower overall profitability. Here are some key findings:
- Emotional Decision-Making: Traders who make decisions based on emotions, rather than data and analysis, are more likely to experience frequent losses.
- Loss Aversion: The fear of loss is a powerful emotion that can cause traders to avoid taking necessary risks, leading to missed opportunities.
- Herding Behavior: The tendency to follow the crowd can be driven by fear or the desire to feel safe, often resulting in poor timing and increased risk.
Strategies for Mastering Emotional Trading
To become a successful trader, it is crucial to develop strategies that help you manage and control your emotions. Here are some practical tips to enhance your emotional resilience and trading performance:
1. Develop a Trading Plan
- Define Your Goals: Clearly outline your financial and personal goals. This helps you stay focused and avoid impulsive decisions.
- Set Rules: Establish specific rules for when to enter and exit trades, and stick to them. This reduces the influence of emotions on your trading decisions.
- Risk Management: Determine the maximum amount of money you are willing to risk on each trade. This helps you maintain discipline and avoid overtrading.
2. Practice Mindfulness and Self-Awareness
- Mindfulness Techniques: Techniques such as meditation, deep breathing, and journaling can help you stay centered and aware of your emotional state.
- Self-Reflection: Regularly reflect on your trading decisions and the emotions that influenced them. This can help you identify patterns and make more rational choices.
3. Maintain a Positive Mindset
- Stay Optimistic: Cultivate a positive attitude towards trading. Believe in your ability to learn and adapt to market conditions.
- Embrace Failure: View losses as opportunities to learn and improve. Every trade, whether a win or a loss, provides valuable insights.
4. Use Technology to Your Advantage
- Automated Trading Systems: Consider using automated trading systems to help you stick to your trading plan and reduce the emotional impact of trading.
- Trading Simulators: Practice on trading simulators to build your confidence and develop your skills without the pressure of real financial stakes.
5. Seek Professional Help
- Therapy and Coaching: If you find it challenging to manage your emotions, consider seeking help from a therapist or a trading coach. They can provide you with tools and techniques to improve your emotional control.
- Support Networks: Join trading communities or forums where you can share experiences and learn from other traders.
The Role of Psychology in Successful Trading
Psychology plays a crucial role in successful trading. By understanding the psychological aspects of trading, you can develop a more disciplined and rational approach. Here are some key psychological principles to keep in mind:
Cognitive Biases
- Confirmation Bias: The tendency to seek out information that confirms your existing beliefs and ignore contradictory evidence.
- Anchoring Bias: The tendency to rely too heavily on the first piece of information you receive, even if it is no longer relevant.
- Hindsight Bias: The tendency to believe, after an event has occurred, that you predicted it before it happened.
Emotional Regulation
- Stress Management: Learn techniques to manage stress, such as exercise, meditation, and proper sleep.
- Emotional Intelligence: Develop your emotional intelligence to better understand and manage your emotions.
- Resilience: Build resilience to bounce back from setbacks and maintain a positive outlook.
Current Data and Research
Recent research highlights the importance of psychological factors in trading. A study by the National Bureau of Economic Research found that traders who are more emotionally stable tend to perform better over the long term. Another study by the University of California, Berkeley, revealed that traders who practice mindfulness have a lower incidence of impulsive decision-making and higher profitability.
Case Studies: Emotionally Resilient Traders
Case Study 1: John Doe
John Doe, a veteran trader, credits his success to his ability to manage his emotions. He follows a strict trading plan, practices mindfulness, and regularly reflects on his trades. John’s disciplined approach has helped him weather market volatility and achieve consistent profits over the years.
Case Study 2: Jane Smith
Jane Smith, a novice trader, struggled with emotional decision-making early in her career. After experiencing significant losses, she sought help from a trading coach and joined a support network. These steps helped her develop a more rational and disciplined trading mindset, leading to improved performance and confidence.
Practical Tips for Emotional Mastery
- Stay Informed: Educate yourself about the psychological aspects of trading. Reading books, attending webinars, and following industry experts can provide valuable insights.
- Keep a Trading Journal: Document your trades, including the emotions you experienced and the lessons you learned. This can help you identify and correct emotional patterns.
- Set Realistic Expectations: Understand that losses are a natural part of trading. Setting realistic expectations can help you maintain a balanced emotional state.
- Take Breaks: Avoid trading when you are feeling stressed or overwhelmed. Taking regular breaks can help you return to trading with a clear and focused mind.
- Stay Physically Healthy: Physical health can significantly impact your emotional well-being. Ensure you get enough sleep, eat well, and exercise regularly.
Conclusion
Mastering the psychological aspects of trading is a critical component of long-term success. By developing a trading plan, practicing mindfulness, maintaining a positive mindset, using technology, and seeking professional help, you can enhance your emotional resilience and improve your trading performance. Remember, the key to mastering emotions is not to eliminate them but to understand and control them. Take the first step today by implementing these strategies and watch your trading journey transform.
Key Takeaway
Emotions are a natural part of trading, but they don’t have to control your decisions. By developing a disciplined approach and enhancing your emotional intelligence, you can make more rational and profitable trades.
Investment Strategies
Unlocking Success with Advanced Portfolio Rebalancing Techniques
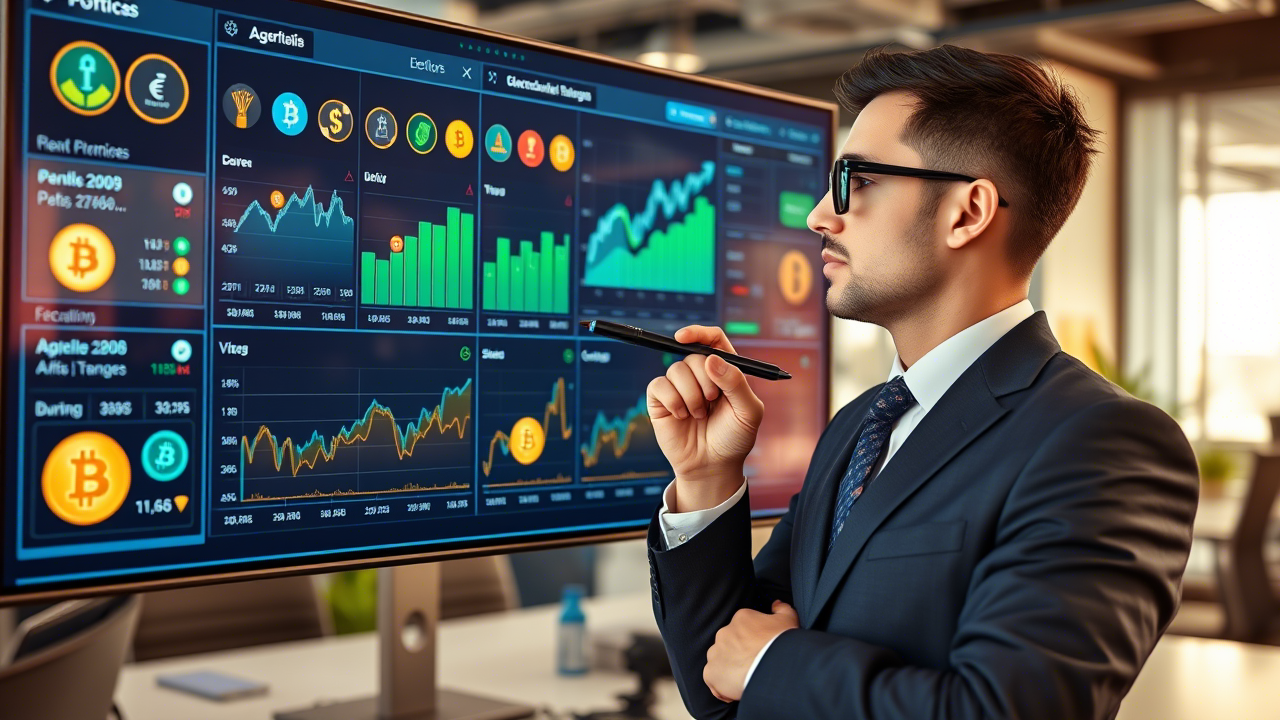

Unlocking Success with Advanced Portfolio Rebalancing Techniques
Introduction
In today’s dynamic financial landscape, investment strategies can become obsolete without proper adjustments. This is where advanced portfolio rebalancing techniques come into play. The process of rebalancing not only helps in maintaining your desired risk level but also in optimizing returns. In this article, we will explore various advanced techniques, current data trends, and practical tips that will enhance your portfolio management skills.
What is Portfolio Rebalancing?
Portfolio rebalancing is the practice of realigning the weightings of assets in a portfolio. This involves periodically buying or selling assets to maintain an original or desired level of asset allocation or risk.
Why is Rebalancing Important?
- Risk Management: Helps to keep the portfolio aligned with your risk tolerance.
- Capital Growth: Encourages disciplined investing which can lead to enhanced returns.
- Emotional Detachment: Reduces the chances of making impulsive investment decisions driven by market emotions.
Key Concepts to Understand
Before diving into advanced techniques, it’s crucial to grasp some key concepts:
- Asset Allocation: The distribution of investments among different asset classes (stocks, bonds, real estate, etc.).
- Risk Tolerance: Your financial ability and willingness to endure fluctuations in your portfolio.
- Investment Horizon: The length of time you expect to hold an investment before taking the funds.
Advanced Portfolio Rebalancing Techniques
1. Time-Based Rebalancing
One of the simplest forms of rebalancing is through the time-based method.
- How It Works: Set a specific time frame (e.g., quarterly, semi-annually) to assess your portfolio.
- Considerations: Market conditions may warrant more frequent rebalancing.
2. Threshold-Based Rebalancing
Threshold-based rebalancing involves setting specific deviation limits for asset classes.
- How It Works: If an asset class exceeds or falls below a predetermined percentage (e.g., 5%), a rebalancing event is triggered.
- Advantages: This method can minimize transaction costs and is less influenced by time intervals.
3. Cash Flow Rebalancing
Utilizing cash flows—such as dividends, interest, or capital from matured bonds—is a seamless method to rebalance.
- How It Works: Instead of selling assets, reinvest new cash inflows to buy underrepresented asset classes.
- Benefits: Avoids transaction costs associated with selling assets and it may provide tax advantages.
4. Risk Parity Rebalancing
This advanced technique focuses on equalizing the risk contributed by each asset class rather than dollar amounts.
- How It Works: Risk is assessed based on the volatility of asset classes. Assets with lower volatility are allocated more capital to equalize risk contribution.
- Outcome: It improves the risk-return profile of a portfolio.
5. Dynamic Rebalancing
This approach involves using quantitative models and algorithms to determine the best time for rebalancing.
- How It Works: Utilizes historical data and market indicators to make real-time adjustments.
- Pros: Adaptability to changing market conditions enables optimized asset allocation.
Data-Driven Insights
- Current Market Trends: In 2023, asset classes like technology stocks have seen high volatility while bonds remain relatively stable, emphasizing the importance of periodic asset evaluation.
- Historical Performance: Studies show that portfolios rebalanced annually tend to perform better over time compared to those left untouched.
- Investor Psychology: Regular rebalancing can mitigate the emotional biases associated with market fluctuations, leading to more rational investment decisions.
Practical Tips for Effective Rebalancing
- Educate Yourself: Stay updated on market trends and financial products.
- Utilize Technology: Consider using robo-advisors or apps designed for portfolio tracking and rebalancing.
- Set Clear Goals: Define your investment objectives and risk tolerance before considering rebalancing techniques.
- Review Regularly: Conduct annual assessments and adjust your strategies as necessary according to changing market conditions.
Conclusion
Implementing advanced portfolio rebalancing techniques can significantly improve your investment performance and align your portfolio with your financial goals. By understanding various approaches—whether time-based, threshold-based, or dynamic—you can establish a strategy that minimizes risk and enhances returns.
Don’t let your investment strategy stagnate; take action today! Assess your current portfolio, determine your risk tolerance, and implement an appropriate rebalancing strategy. With thoughtful management, you can navigate the complexities of today’s markets and emerge as a successful investor.
Call to Action: Start optimizing your portfolio today! Explore our financial resources and tools designed to help you achieve your investment goals.
Investment Strategies
Exploring Arbitrage Opportunities in Crypto Markets
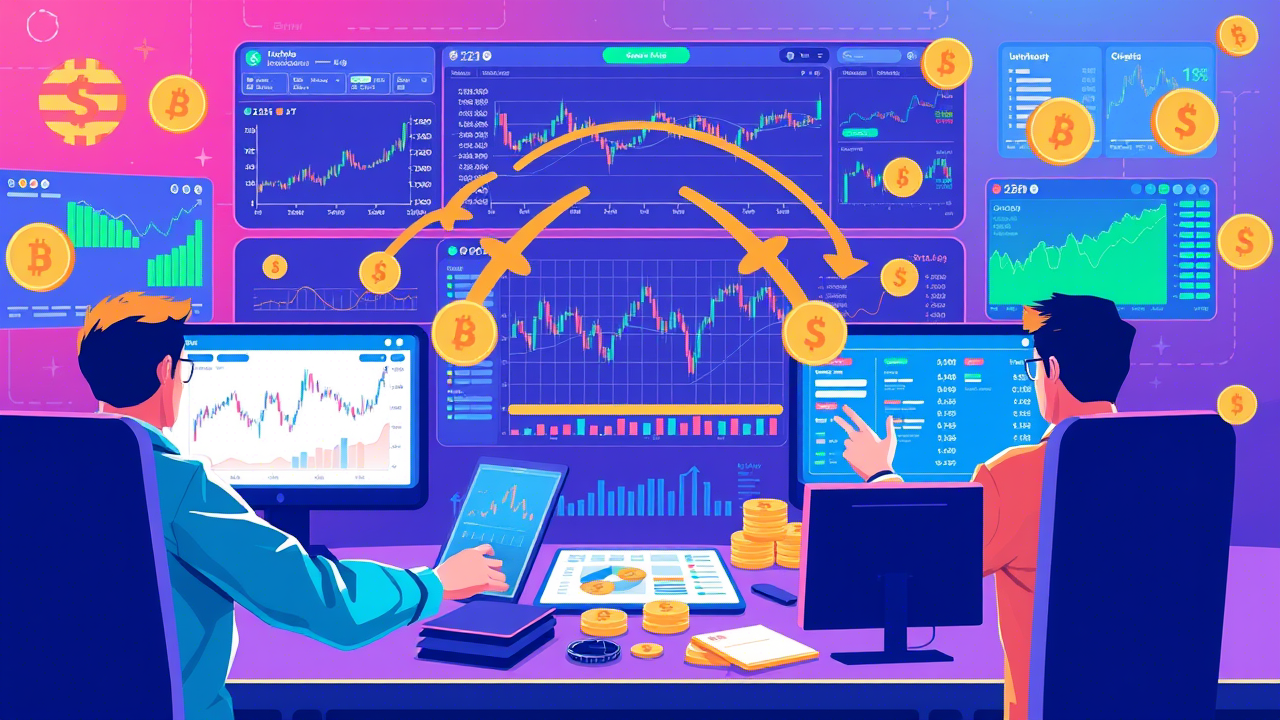

Exploring Arbitrage Opportunities in Crypto Markets
Introduction
The world of cryptocurrency is a tumultuous ocean of volatility, innovation, and opportunity. Among the various trading strategies that crypto enthusiasts employ, exploring arbitrage opportunities in crypto markets stands out as a method that allows traders to leverage price discrepancies across different exchanges. This article will delve into what arbitrage is, the different types of arbitrage in the crypto space, practical tips on how to identify and take advantage of these opportunities, and the tools that can facilitate your trading journey.
Understanding Arbitrage
Arbitrage refers to the practice of taking advantage of price differences of the same asset across different markets. In the realm of cryptocurrency, this could mean buying a coin at a lower price on one exchange and selling it at a higher price on another. Given the fragmented nature of crypto markets, price inconsistencies are common and can be exploited.
Types of Arbitrage in Crypto Markets
- Spatial Arbitrage:
- This is the most common form, where traders buy cryptocurrency on one exchange and simultaneously sell it on another. The key is speed; the quicker you execute the trade, the better your chances of profiting from the price difference.
- Triangular Arbitrage:
- This involves trading between three different currencies on a single exchange. Traders exploit the currency conversion rates to find profit opportunities that might exist in the inter-trade rates.
- Statistical Arbitrage:
- This is a more complex approach that uses mathematical models and statistical methods. Traders focus on historical price movements and correlations between various cryptocurrencies.
- Decentralized Exchange Arbitrage:
- With the rise of decentralized finance (DeFi), many traders look for arbitrage opportunities between centralized exchanges and decentralized exchanges. As these markets often have different liquidity levels, price discrepancies can emerge.
Identifying Arbitrage Opportunities
Tools and Resources
To effectively exploit arbitrage opportunities in the crypto markets, you need the right tools at your disposal:
- Arbitrage Bots: These automated tools can monitor price differences across exchanges 24/7, executing trades faster than a human trader could.
- Price Aggregator Websites: Websites like CoinMarketCap or CoinGecko can provide real-time price updates across multiple exchanges, helping you spot price discrepancies quickly.
- Trading View Platforms: Tools like TradingView can help you analyze market trends and price movements visually, adding another layer to your decision-making process.
Key Factors to Consider
- Transaction Fees:
- Always factor in transaction fees when calculating potential profits. Sometimes the fees can erode your gains from arbitrage activities.
- Market Liquidity:
- Ensure the markets you are trading in have sufficient liquidity. Higher liquidity often results in better execution prices.
- Exchange Trustworthiness:
- Not all exchanges are created equal. Opt for reputable exchanges with good security measures in place to avoid losses due to hacks or insolvency.
- Speed of Execution:
- In arbitrage, time is of the essence. Utilize tools that facilitate quick transactions, as the market can change rapidly.
Practical Steps to Get Started
- Choose Your Exchanges:
- Start by selecting a few exchanges with significant volume and liquidity. Familiarize yourself with their trading interfaces and withdrawal processes.
- Set Up Accounts:
- Ensure you have your accounts set up and verified on all selected exchanges to facilitate quick trading operations.
- Develop a Trading Strategy:
- Outline how you’ll identify opportunities, conduct transactions, and manage risk. Consider using stop-loss orders to minimize potential losses.
- Monitor the Markets:
- Regularly check the price differences across your selected exchanges. Use alerts to notify you when price discrepancies exceed a certain threshold.
- Start Small:
- Initially, conduct a few trades with a small amount of capital. This will allow you to gauge the effectiveness of your strategy without risking significant amounts of money.
Common Risks and Challenges
- Market Volatility: Price fluctuations can occur quickly, eroding potential arbitrage gains before a trader can execute a transaction.
- Regulatory Risks: Stay abreast of regulations in your jurisdiction, as they can impact your trading activities.
- Exchange Downtime: Technical issues or maintenance on exchanges can prevent you from executing trades when opportunities arise.
Conclusion
Exploring arbitrage opportunities in crypto markets can be a lucrative venture for traders who are equipped with the right knowledge, tools, and strategies. While it’s not without its risks, understanding the nuances of the market can help you navigate through volatility and price discrepancies. As with any trading strategy, ongoing education and vigilance will be your allies as you delve into arbitrage trading.
Call to Action
Are you ready to dive into the exciting world of crypto arbitrage? Start by researching the exchanges, setting up your accounts, and preparing to seize those fleeting opportunities. The next price disparity could make all the difference in your trading success!
Investment Strategies
The Basics of Dollar-Cost Averaging (DCA) in Crypto: A Smart Investment Strategy
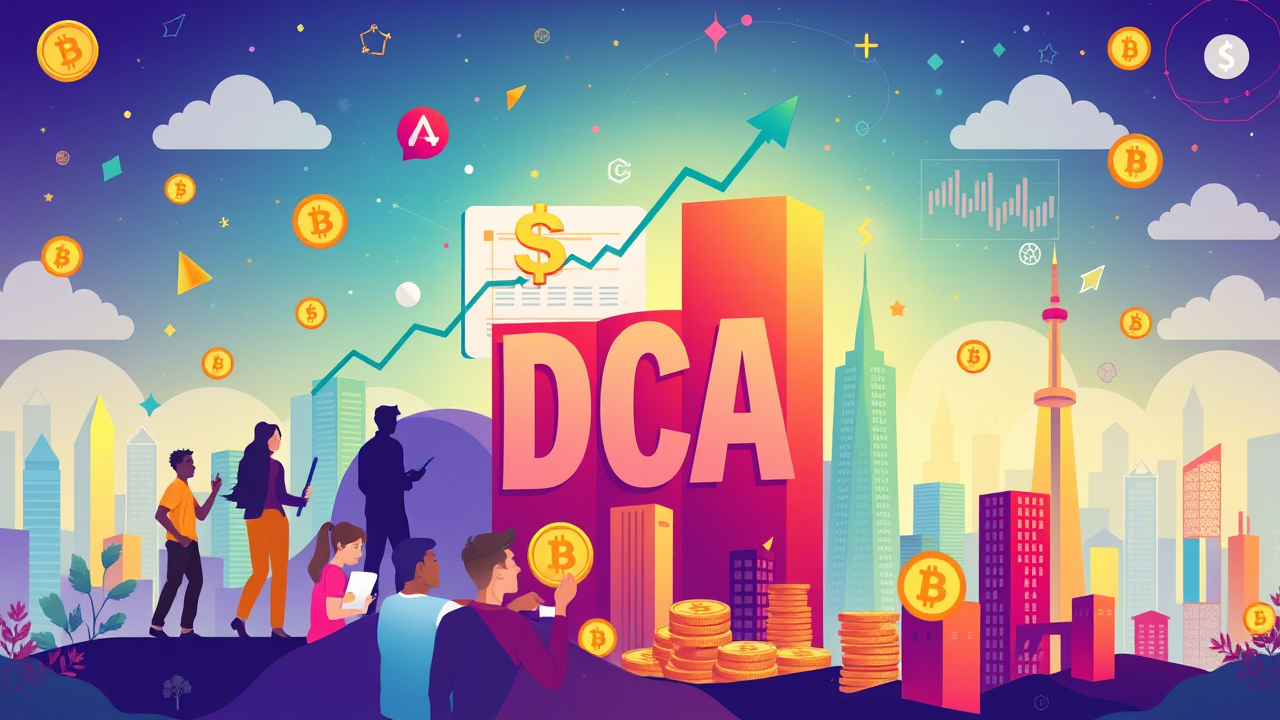

The Basics of Dollar-Cost Averaging (DCA) in Crypto
Introduction
Cryptocurrency is highly volatile, making it a challenging landscape for investors at all levels. Enter Dollar-Cost Averaging (DCA), a strategy that can provide a systematic and less stressful approach to buying crypto assets. In this article, we will delve into the basics of DCA in crypto, its benefits, potential drawbacks, and practical tips to get started.
What is Dollar-Cost Averaging (DCA)?
Dollar-Cost Averaging is an investment strategy that involves regularly investing a fixed amount of money into a particular asset, regardless of its price. This approach promotes financial discipline and helps mitigate the effects of market volatility. In the world of cryptocurrency, DCA can be especially beneficial given the rapid price fluctuations common in this space.
How DCA Works
- Fixed Amount Investment: Decide on a fixed amount you want to invest. This could be weekly, monthly, or quarterly based on your financial situation.
- Consistent Purchasing: Purchase crypto at regular intervals, irrespective of the current price.
- Long-Term Strategy: Keep a long-term view, as DCA works best with time, allowing you to take advantage of market dips and rises.
Example of DCA in Crypto
Suppose you decide to invest $100 each month in Bitcoin. Whether Bitcoin trades at $40,000, $35,000, or $50,000, you will buy Bitcoin worth $100 every month. Over time, you’ll accumulate Bitcoin at an average price, which helps to mitigate the risk of market timing.
Benefits of Using DCA in Crypto
- Reduces Emotional Trading: DCA can help alleviate trading anxiety related to the volatile nature of crypto. It encourages consistent investing without emotional reactions to market fluctuations.
- Mitigates Market Timing Risk: Timing the market is notoriously difficult. By investing a fixed amount regularly, you spread out your entry points, helping to reduce the overall impact of price volatility.
- Encourages Discipline: A consistent approach fosters good investment habits. Whether the market is up or down, you’re following a plan.
- Simplifies Investments: For beginners, DCA offers a straightforward strategy that avoids the complexities of market analysis.
- Long-Term Growth: DCA is ideal for a long-term investment perspective. By accumulating assets over time, you can benefit from compounding interest and overall growth.
Potential Drawbacks of DCA
While DCA has numerous advantages, it is essential to recognize its potential drawbacks:
- Missed Opportunities: In a bullish market, lump-sum investments may outperform DCA since you could purchase more at lower prices.
- Transaction Fees: If your trading platform charges fees per transaction, frequent buying could lead to increased overall costs. Be sure to consider the implications of fees.
- Required Discipline: While DCA can be beneficial, maintaining discipline over many months or years can be challenging, especially during market downturns.
Practical Tips for Implementing DCA in Crypto
1. Choose Your Crypto Wisely
Research various cryptocurrencies and select those that have strong fundamentals, community support, and a good use case. Bitcoin and Ethereum are popular choices for beginners, but consider diversifying your portfolio with other promising altcoins.
2. Set a Budget
Decide how much you can afford to invest each month without affecting your living expenses. Start small if needed and gradually increase your investment as you become more comfortable.
3. Select the Right Interval
Determine how frequently you want to invest. Monthly is a common choice, but you can adjust this based on market conditions or personal preference.
4. Use Automated Strategies
Many platforms offer automated DCA strategies that will execute your trades on your behalf at set intervals. Platforms like Coinbase, Binance, and Kraken provide these services, making investing simpler.
5. Monitor Your Investments
Even with DCA, it’s essential to regularly review your portfolio’s performance. Adjust your strategy as necessary based on market trends and your long-term financial goals.
6. Stay Educated
Cryptocurrency markets evolve rapidly. Keep yourself informed with current data, updates on specific cryptocurrencies, and market trends. Knowledge can empower you to make informed decisions.
7. Have a Long-Term Perspective
DCA is not a get-rich-quick scheme. Stay committed to your strategy and remember to keep your long-term goals in sight.
Conclusion
Dollar-Cost Averaging is a practical strategy that can help mitigate risks associated with the volatile nature of cryptocurrency investing. By allowing you to invest consistently over time, DCA reduces the impact of market fluctuations and promotes disciplined investing.
With the right approach, DCA can enhance your crypto portfolio and make your trading journey less daunting. As with any investment strategy, it’s crucial to perform thorough research and continually educate yourself about the cryptocurrency market. Start incorporating DCA into your investment plan today, and watch as it transforms your financial journey.
Call to Action: Ready to take your first step into the world of cryptocurrency investing with Dollar-Cost Averaging? Sign up with an exchange like Coinbase or Binance and start building your portfolio today!
-
Security & Privacy1 week ago
Advanced Techniques for Securing Multi-Signature Wallets
-
Crypto Basics1 week ago
How to Spot Fake News in the Crypto Space: A Comprehensive Guide for Savvy Investors
-
Regulations & Policy Updates7 days ago
Legal Frameworks for Launching Security Tokens: A Comprehensive Guide for 2025
-
Blockchain Education5 days ago
What Is Blockchain Technology? A Beginner-Friendly Explanation
-
Web3 & Metaverse7 days ago
Title: Implementing Zero-Knowledge Proofs in Web3 Applications: A Comprehensive Guide to Security and Privacy
-
Video1 week ago
Public vs Private Blockchain | Difference Between Public and Private Blockchain
-
Crypto Basics1 week ago
Breaking Down the Latest Crypto Regulations Around the World: Insights, Trends, and Practical Tips
-
Video5 days ago
Top Mistakes New Investors Make in Crypto